Computer Engineering
Computer Engineering in Heidelberg has links to many research fields in the university where large amounts of data must be acquired or processed quickly. ZITI has the competences to develop electronics microchips for specific applications in sensing or data transport from scratch. Data communication being one of the largest sources of power consumption, is addressed on the hardware level, by hardware aware programming and adapted system design. Apart from efficient programming of modern processors, ZITI operates a variety of coprocessors (FPGA, GPU, ML-coprocessors) in order to exploit their specific strengths and research methods and workflows for most efficient data processing.
Circuit Design
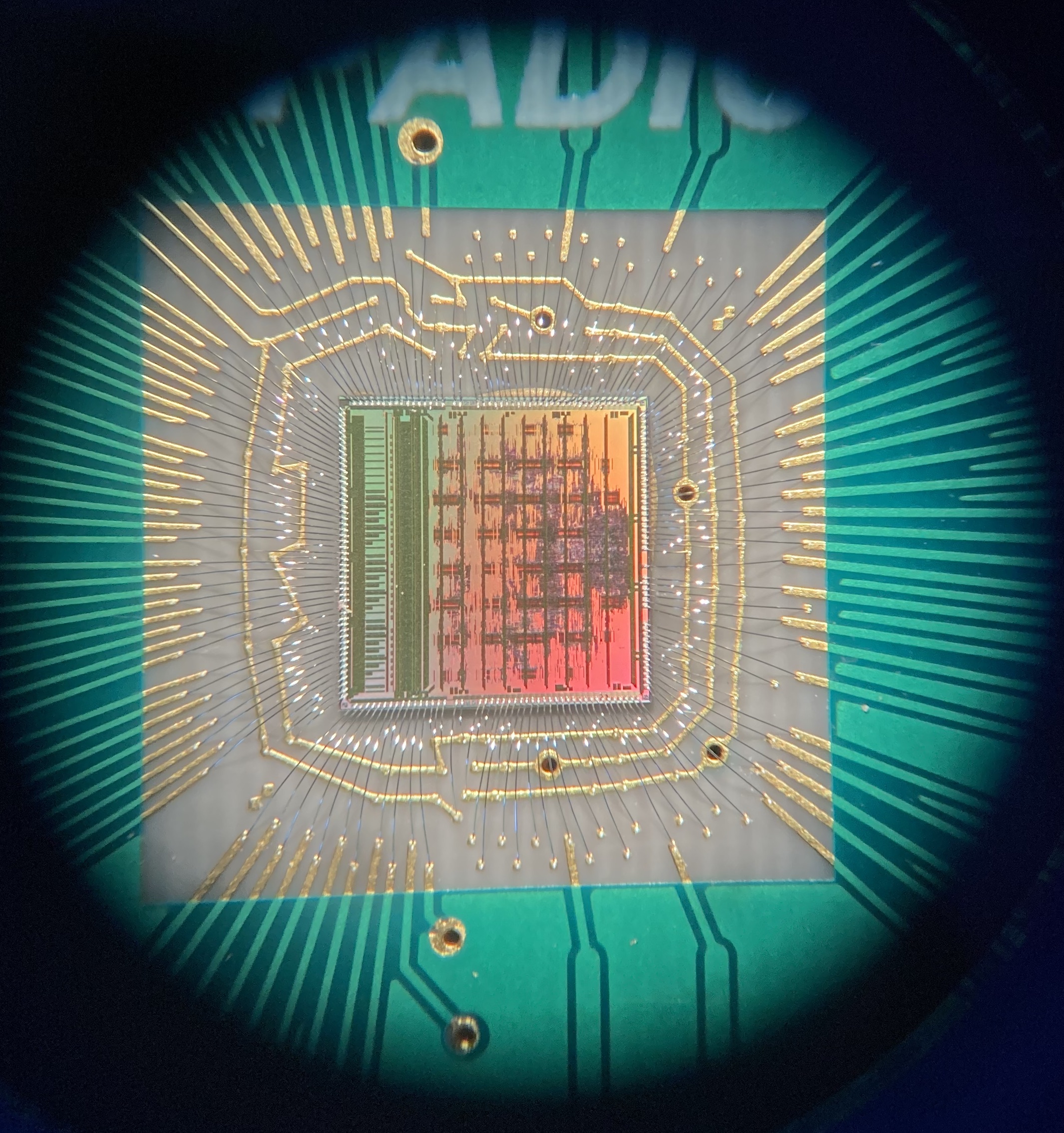
At the chair of Circuit Design, microelectronic circuits are developed, tested and applied. These microchips often contain extremely sensitive, low noise amplifiers for capturing sensor data and blocks for further analog and digital signal processing. Some chips contain particle- or (single) photon-sensitive structures. The crucial analogue parts of such chips are designed completely manually. Complex digital blocks described by HDL languages are converted to a layout by a suitable tool chain and both parts are merged. The chips are fabricated in state-of-the-art CMOS technologies and commissioned by suited additional hardware (often FPGA boards) in the group.
Examples projects are readout and processing chips for particle detectors (e.g. see picture), single photon sensors for dark matter search or microscopy, hybrid pixel detectors for synchrotron X-ray detection at Eu-XFEL or ESRF or chips for state-of-the-art PET scanners.
Computing Systems
Today, research in computing systems is most concerned with specialized forms of computing in combination with seamless integration into existing systems. Specialized computing, for instance based on GPUs (as known for gaming) or FPGAs (field programmable gate arrays) or ASICs (not the shoe brand but “application-specific integrated circuits”), is motivated by diminishing returns from CMOS technology scaling and hard power constraints. Notably, for a given fixed power budget , energy efficiency defines performance : . Thus, a sustained performance scaling based on CMOS technology requires to improve the energy efficiency of compute and memory operations substantially, which is typically being done using the previously mentioned specialized forms of computing. However, any specialization stands in contrast to generality, thus raising various questions related to programmability and algorithmic innovation.
Particular research foci include
- resource-efficient ML such as model compression for edge, mobile and embedded systems
- code analysis and generation as for instance based on CLANG/LLVM and targeting (multi-)GPU systems
- HW/SW codesign to meet application objectives by a comprehensive treatment of software and hardware components
- specialized processor architectures under performance, energy efficiency and programmability constraints
The group is most concerned with bridging the gap in between application and hardware, including automated tools as well as abstract models that facilitate reasoning about various optimizations and decisions.
Application Specific Computing
Our research focuses on significant improvements of performance and accuracy in application specific computing through a global optimization across the entire spectrum of numerical methods, algorithm design, software implementation and hardware acceleration.
These layers typically have contradictory requirements and their integration poses many challenges. For example, numerically superior methods expose little parallelism, bandwidth efficient algorithms convolve the processing of space and time into unmanageable software patterns, high level language abstractions create data layout and composition barriers, and high performance on today's hardware poses strict requirements on parallel execution and data access. High performance and accuracy for the entire application can only be achieved by balancing these requirements across all layers.
The following topics are given particular attention:
- Data representation (mixed-precision, compression, redundancy)
- Data access (layout, spatial and temporal locality)
- Data structure (unstructured grids, graphs, adaptivity)
- Numerical methods (ILU, Krylov, GMG, AMG)
- Programming abstractions (CUDA, thrust, PSTL, C++2x, UPC++)
Research Group Leaders
Design of Microelectronic circuits and sensor systems for particle and photon detection
Future and emerging technology (GPUs, FPGAs, ASICs, resistive RAM) for high-performance computing, resource-constrained machine learning and data analytics
Parallel algorithms and hardware (GPU, many-core CPU, FPGA) in relation to data representation, data access, data structure, numerical methods and programming abstractions